Computer-Aided Diagnosis for the Early Breast Cancer Detection
DOI:
https://doi.org/10.21928/uhdjst.v7n1y2023.pp7-14Keywords:
Computer-aided Diagnosis, Medical Image, Breast Cancer, Gray Level Run Length Matrix, Classifier TechniqueAbstract
The development of the use of medical image processing in the healthcare sector has contributed to enhancing the quality/accuracy of disease diagnosis or early detection because diagnosing a disease or cancer and identifying treatments manually is costly, time-consuming, and requires professional staff. Computer-aided diagnosis (CAD) system is a prominent tool for the detection of different forms of diseases, especially cancers, based on medical imaging. Digital image processing is a critical in the processing and analysis of medical images for the disease diagnosis and detection. This study introduces a CAD system for detecting breast cancer. Once the breast region is segmented from the mammograms image, certain texture and statistical features are extracted. Gray level run length matrix feature extraction technique is implemented to extracted texture features. On the other hand, statistical features such as skewness, mean, entropy, and standard deviation are extracted. Consequently, on the basis of the extracted features, support vector machine and K-nearest neighbor classifier techniques are utilized to classify the segmented region as normal or abnormal. The performance of the proposed approach has been investigated through extensive experiments conducted on the well-known Mammographic Image Analysis Society dataset of mammography images. The experimental findings show that the suggested approach outperforms other existing approaches, with an accuracy rate of 99.7%.
References
A. A. Abdulla. “Efficient computer-aided diagnosis technique for leukaemia cancer detection”. The Institution of Engineering and Technology, vol. 14, no. 17, pp. 4435-4440, 2020.
A. A. Abdulla and M. W. Ahmed. “An improved image quality algorithm for exemplar-based image inpainting”. Multimedia Tools and Applications, vol. 80, pp. 13143-13156, 2021.
H. Arimura, T. Magome, Y. Yamashita and D. Yamamoto. “Computer-aided diagnosis systems for brain diseases in magnetic resonance images”. Algorithms, vol. 2, no. 3, pp. 925-952, 2009.
G. Kumar and P. K. Bhatia. “A Detailed Review of Feature Extraction in Image Processing Systems”. International Conference on Advanced Computing and Communication Technologies ACCT, pp. 5-12, 2014.
T. T. Htay and S. S. Maung. “Early Stage Breast Cancer Detection System Using GLCM Feature Extraction and K-Nearest Neighbor (k-NN) on Mammography Image”. 2018-The 18th International Symposium on Communications and Information Technologies, pp. 345-348, 2018.
Centers for Disease Control and Prevention. “What Is Breast Cancer?”. Centers for Disease Control and Prevention, United States. 2021. Available from: https://www.cdc.gov/cancer/breast/ basic_info/what-is-breast-cancer.html [Last accessed on 2022 Dec 18].
J. Vasković. “Overview and Types of Connective Tissue.” Medical and Anatomy Experts, 2022. Available from: https://www.kenhub. com/en/library/anatomy/overview-and-types-of-connective-tissue [Last accessed on 2022 Dec 20].
M. M. Eltoukhy, I. Faye and B. B. Samir. “Breast cancer diagnosis in digital mammogram using multiscale curvelet transform”. Computerized Medical Imaging and Graphics, vol. 34, no. 4, pp. 269-276, 2010.
S. Srivastava, N. Sharma, S. K. Singh and R. Srivastava. “Design, analysis and classifier evaluation for a CAD tool for breast cancer detection from digital mammograms”. International Journal of Biomedical Engineering and Technology, vol. 13, no. 3, pp. 270- 300, 2013.
S. C. Satapathy, B. N. Biswal, S. K. Udgata and J. K. Mandal. “Proceedings of the 3rd International Conference on Frontiers of Intelligent Computing: Theory and Applications (FICTA) 2014”. Advances in Intelligent Systems and Computing, vol. 327, pp. 413-419, 2014.
S. Pashoutan, S. B. Shokouhi and M. Pashoutan. “Automatic Breast Tumor Classification Using a Level Set Method and Feature Extraction in Mammography.” 2017 24th Iranian Conference on Biomedical Engineering and 2017 2nd International Iranian Conference on Biomedical Engineering ICBME 2017, pp. 1-6, 2018.
V. Hariraj, W. Khairunizam, V. Vijean and Z. Ibrahim. “Fuzzy multi-layer SVM classification”. International Journal of Mechanical Engineering and Technology (IJMET), vol. 9, pp. 1281-1299, 2018.
S. J. A. Sarosa, F. Utaminingrum and F. A. Bachtiar. “Breast cancer classification using GLCM and BPNN”. International Journal of Advances in Soft Computing and Its Applications, vol. 11, no. 3, pp. 157-172, 2019.
A. Arafa, N. El-Sokary, A. Asad and H. Hefny. “Computer-aided detection system for breast cancer based on GMM and SVM”. Arab Journal of Nuclear Sciences and Applications, vol. 52, no. 2, pp. 142-150, 2019.
A. H. Farhan and M. Y. Kamil. “Texture analysis of breast cancer via LBP, HOG, and GLCM techniques”. IOP Conference Series: Materials Science and Engineering, vol. 928, no. 7, p. 072098, 2020.
A. S. Eltrass and M. S. Salama. “Fully automated scheme for computer-aided detection and breast cancer diagnosis using digitised mammograms”. IET The Institution of Engineering and Technology, vol. 14, no. 3, pp. 495-505, 2020.
E. M. H. Saeed, H. A. Saleh and E. A. Khalel. “Classification of mammograms based on features extraction techniques using support vector machine”. Computer Science and Information Technologies, vol. 2, no. 3, pp. 121-131, 2020.
H. Mu’jizah and D. C. R. Novitasari. “Comparison of the histogram of oriented gradient, GLCM, and shape feature extraction methods for breast cancer classification using SVM”. Journal of Technology and Computer Systems, vol. 9, no. 3, pp. 150-156, 2021.
G. Holi. “Automatic breast cancer detection with optimized ensemble of classifiers”. International Journal of Advanced Research in Engineering and Technology (IJARET), vol. 11, no. 11, pp. 2545-2555, 2020.
V. R. Nwadike. “What Does Breast Cancer Look Like on a Mammogram?”. 2018. Available from: https://www. medicalnewstoday.com/articles/322068 [Last accessed on 2022 Dec 16].
K. Bhargavi and S. Jyothi. “A survey on threshold based segmentation technique in image processing”. International Journal of Innovative Research and Development, vol. 3, no. 12, pp. 234-239, 2014.
J. Suckling, J. Parker, D. Dance, S. Astley, I. Hutt, C. Boggis, I. Ricketts, E. Stamatakis, N. Cerneaz, N, S. Kok, P. Taylor, D. Betal and J. Savage. “The mammographic image analysis society digital mammogram database”. International Congress Series, vol. 1069, pp. 375-378, 1994.
R. Murtirawat, S. Panchal, V. K. Singh and Y. Panchal. “Breast Cancer Detection Using K-Nearest Neighbors, Logistic Regression and Ensemble Learning”. Proceedings of the International Conference on Electronics and Sustainable Communication Systems, ICESC 2020, pp. 534-540, 2020.
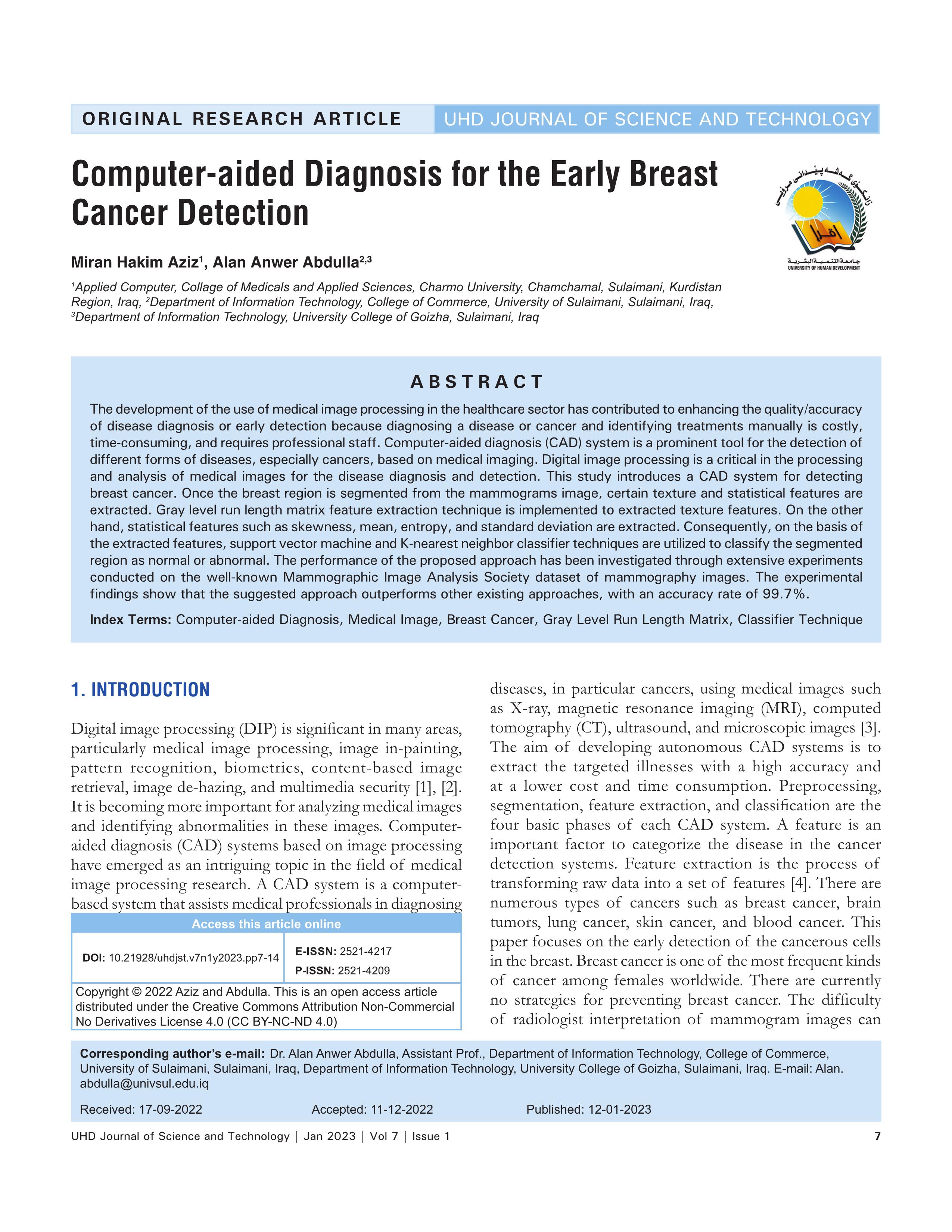
Downloads
Published
How to Cite
Issue
Section
License
Copyright (c) 2023 Miran Hakim Aziz, Alan Anwer Abdulla

This work is licensed under a Creative Commons Attribution-NonCommercial-NoDerivatives 4.0 International License.